Research Trends on Machine Learning in Construction Management: A Scientometric Analysis
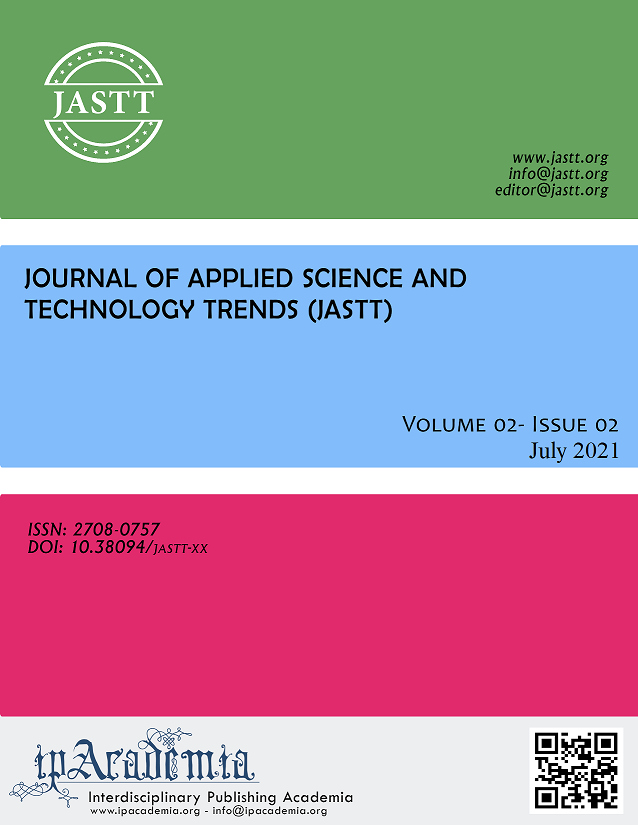
Abstract
Machine learning plays a vital role in construction industry which could make improve project’s safety, productivity, and quality. Many studies have attempted to explore the potential opportunities to adopt this technology in different aspects of the construction sector. However, no comprehensive study to review the global research trends on this technological advancement in construction management domain. The goal is to investigate and summarize the state-of-the-art knowledge body in this topic in a systematic manner. To achieve this, this paper considered 161 studies on machine learning in construction management related to bibliographic records retrieved from the Scopus database by adopting scientometric analysis approach. This paper found that since 2014, there has been a considerable increase in the number of publications on this domain. Researchers from the United States, China, and Australia have been the main contributors to this research area through regional analysis. This study also revealed that approximately 34% of all countries in the world are engaged in this domain research. In addition, five main aspects in construction management have been applied machine learning techniques, namely, assess and reduce risk, safety management for construction sites, cost estimation and prediction, Schedule management, and building energy demand prediction. Furthermore, three potential construction management research areas that can apply this technology were proposed for further studies. The findings will help both professionals and researchers more understanding how machine learning knowledge is evolving and its role played in the construction management domain, and this study thus offers a useful reference point to how can develop this area in the future.
Keywords
Machine Learning, Construction Management, Scientometric
References
- Akinosho, T.D., et al., Deep learning in the construction industry: A review of present status and future innovations. Journal of Building Engineering, 2020: p. 101827.
- Thompson, N., et al., Digitalisation in Construction-Industrial Strategy Review, supporting the Government's Industrial Strategy. University College London, London, 2017.
- Van Tam, N., et al., Impact of BIM-related factors affecting construction project performance. International Journal of Building Pathology and Adaptation, 2021. ahead-of-print(ahead-of-print) https://doi.org/10.1108/IJBPA-05-2021-0068
- Van Tam, N., N.L. Huong, and N.B. Ngoc, Factors affecting labour productivity of construction worker on construction site: A case of Hanoi. Journal of Science and Technology in Civil Engineering (STCE)-NUCE, 2018. 12(5): p. 127-138. https://doi.org/10.31814/stce.nuce2018-12(5)-13
- Van Tam, N., et al., Factors affecting adoption of building information modeling in construction projects: A case of Vietnam. Cogent Business & Management, 2021. 8(1): p. 1918848, https://doi.org/10.1080/23311975.2021.1918848
- Lund, D., et al., Worldwide and regional internet of things (iot) 2014–2020 forecast: A virtuous circle of proven value and demand. International Data Corporation (IDC), Tech. Rep, 2014. 1(1): p. 9.
- Hegde, J. and B. Rokseth, Applications of machine learning methods for engineering risk assessment–A review. Safety science, 2020. 122: p. 104492.
- Ajayi, A., et al., Deep Learning Models for Health and Safety Risk Prediction in Power Infrastructure Projects. Risk Analysis, 2020. 40(10): p. 2019-2039.
- Antwi-Afari, M.F., et al., Construction Activity Recognition and Ergonomic Risk Assessment Using a Wearable Insole Pressure System. Journal of Construction Engineering and Management, 2020. 146(7).
- Fan, C.L., Defect Risk Assessment Using a Hybrid Machine Learning Method. Journal of Construction Engineering and Management, 2020. 146(9).
- Gondia, A., et al., Machine Learning Algorithms for Construction Projects Delay Risk Prediction. Journal of Construction Engineering and Management, 2020. 146(1).
- Hsu, M.F., Integrated multiple-attribute decision making and kernel-based mechanism for risk analysis and evaluation. Journal of Intelligent and Fuzzy Systems, 2019. 36(3): p. 2895-2905.
- Kifokeris, D. and Y. Xenidis, Risk source-based constructability appraisal using supervised machine learning. Automation in Construction, 2019. 104: p. 341-359.
- Liu, H. and G. Tian, Building engineering safety risk assessment and early warning mechanism construction based on distributed machine learning algorithm. Safety Science, 2019. 120: p. 764-771.
- Nasir, M., et al., A comparative data analytic approach to construct a risk trade-off for cardiac patients’ re-admissions. Industrial Management and Data Systems, 2019. 119(1): p. 189-209.
- Sanni-Anibire, M.O., R.M. Zin, and S.O. Olatunji, Machine learning model for delay risk assessment in tall building projects. International Journal of Construction Management, 2020.
- Wang, F., et al., Modeling tunnel construction risk dynamics: Addressing the production versus protection problem. Safety Science, 2016. 87: p. 101-115.
- Yang, B., Construction of logistics financial security risk ontology model based on risk association and machine learning. Safety Science, 2020. 123.
- Poh, C.Q., C.U. Ubeynarayana, and Y.M. Goh, Safety leading indicators for construction sites: A machine learning approach. Automation in construction, 2018. 93: p. 375-386.
- Asadzadeh, A., et al., Sensor-based safety management. Automation in Construction, 2020. 113.
- Goh, Y.M. and N.F. Binte Sa’adon, Cognitive factors influencing safety behavior at height: a multimethod exploratory study. Journal of Construction Engineering and Management, 2015. 141(6): p. 04015003.
- Goh, Y.M. and D. Chua, Neural network analysis of construction safety management systems: a case study in Singapore. Construction Management and Economics, 2013. 31(5): p. 460-470.
- Gong, P., et al., Safety risk evaluations of deep foundation construction schemes based on imbalanced data sets. Journal of Civil Engineering and Management, 2020. 26(4): p. 380-395.
- Harirchian, E., et al., A machine learning framework for assessing seismic hazard safety of reinforced concrete buildings. Applied Sciences (Switzerland), 2020. 10(20): p. 1-18.
- Hou, L., et al., Deep learning-based applications for safety management in the AEC industry: A review. Applied Sciences (Switzerland), 2021. 11(2): p. 1-18.
- Lee, S.H. and J. Son, Development of a safety management system tracking the weight of heavy objects carried by construction workers using fsr sensors. Applied Sciences (Switzerland), 2021. 11(4): p. 1-15.
- Seong, H., H. Son, and C. Kim, A Comparative Study of Machine Learning Classification for Color-based Safety Vest Detection on Construction-Site Images. KSCE Journal of Civil Engineering, 2018. 22(11): p. 4254-4262.
- Zhou, Z., J. Irizarry, and Q. Li, Using network theory to explore the complexit of subway construction accident network (SCAN) for promoting safety management. Safety Science, 2014. 64: p. 127-136.
- Tixier, A.J.-P., et al., Application of machine learning to construction injury prediction. Automation in construction, 2016. 69: p. 102-114.
- Hashemi, S.T., O.M. Ebadati, and H. Kaur, Cost estimation and prediction in construction projects: A systematic review on machine learning techniques. SN Applied Sciences, 2020. 2(10): p. 1-27.
- Ji, S.-H., et al., Cost estimation model using modified parameters for construction projects. Advances in Civil Engineering, 2019. 2019.
- Khalaf, T.Z., et al., Particle swarm optimization based approach for estimation of costs and duration of construction projects. Civil Engineering Journal, 2020. 6(2): p. 384-401.
- Jiang, Q., Estimation of construction project building cost by back-propagation neural network. Journal of Engineering, Design and Technology, 2019.
- Chandanshive, V. and A.R. Kambekar, Estimation of building construction cost using artificial neural networks. Journal of Soft Computing in Civil Engineering, 2019. 3(1): p. 91-107.
- Wang, X., Application of fuzzy math in cost estimation of construction project. Journal of Discrete Mathematical Sciences and Cryptography, 2017. 20(4): p. 805-816.
- Du, Z. and B. Li. Construction project cost estimation based on improved BP Neural Network. in 2017 International Conference on Smart Grid and Electrical Automation (ICSGEA). 2017. IEEE.
- Alshemosi, A.M.B. and H.S.H. Alsaad, Cost estimation process for construction residential projects by using multifactor linear regression technique. Int J Sci Res, 2017. 6(6): p. 151-156.
- Bala, K., S.A. Bustani, and B.S. Waziri, A computer-based cost prediction model for institutional building projects in Nigeria. Journal of Engineering, Design and Technology, 2014.
- He, X., et al. Cost estimation of construction project using fuzzy neural network model embedded with modified particle optimizer. in Advanced materials research. 2011. Trans Tech Publ.
- Arafa, M. and M. Alqedra, Early stage cost estimation of buildings construction projects using artificial neural networks. Journal of Artificial Intelligence, 2011. 4(1).
- Rafiei, M.H. and H. Adeli, Novel machine-learning model for estimating construction costs considering economic variables and indexes. Journal of construction engineering and management, 2018. 144(12): p. 04018106.
- Hegazy, T. and A. Ayed, Neural network model for parametric cost estimation of highway projects. Journal of construction engineering and management, 1998. 124(3): p. 210-218.
- Mahalakshmi, G. and C. Rajasekaran, Early cost estimation of highway projects in India using artificial neural network, in Sustainable construction and building materials. 2019, Springer. p. 659-672.
- Kim, S., Hybrid forecasting system based on case-based reasoning and analytic hierarchy process for cost estimation. Journal of Civil Engineering and Management, 2013. 19(1): p. 86-96.
- Chou, J.-S., Generalized linear model-based expert system for estimating the cost of transportation projects. Expert Systems with Applications, 2009. 36(3): p. 4253-4267.
- Shahandashti, S. and B. Ashuri, Highway construction cost forecasting using vector error correction models. Journal of management in engineering, 2016. 32(2): p. 04015040.
- Wilmot, C.G. and B. Mei, Neural network modeling of highway construction costs. Journal of construction engineering and management, 2005. 131(7): p. 765-771.
- Sodikov, J., Cost estimation of highway projects in developing countries: artificial neural network approach. Journal of the Eastern Asia Society for Transportation Studies, 2005. 6: p. 1036-1047.
- Alshamrani, O.S., Construction cost prediction model for conventional and sustainable college buildings in North America. Journal of Taibah University for Science, 2017. 11(2): p. 315-323.
- Hyari, K.H., A. Al-Daraiseh, and M. El-Mashaleh, Conceptual cost estimation model for engineering services in public construction projects. Journal of Management in Engineering, 2016. 32(1): p. 04015021.
- Yu, W.D., PIREM: a new model for conceptual cost estimation. Construction management and economics, 2006. 24(3): p. 259-270.
- Skitmore, R.M. and S.T. Ng, Forecast models for actual construction time and cost. Building and environment, 2003. 38(8): p. 1075-1083.
- Bowen, P. and P. Edwards, Cost modelling and price forecasting: practice and theory in perspective. Construction Management and Economics, 1985. 3(3): p. 199-215.
- Tijanic, K., D. Car-Pusic, and M. Sperac, Cost estimation in road construction using artificial neural network. Neural Computing and Applications, 2019: p. 1-13.
- Swei, O., J. Gregory, and R. Kirchain, Construction cost estimation: A parametric approach for better estimates of expected cost and variation. Transportation Research Part B: Methodological, 2017. 101: p. 295-305.
- Pesko, I., et al., Estimation of costs and durations of construction of urban roads using ANN and SVM. Complexity, 2017. 2017.
- Chou, J.-S., Cost simulation in an item-based project involving construction engineering and management. International Journal of Project Management, 2011. 29(6): p. 706-717.
- Kim, K.J. and K. Kim, Preliminary cost estimation model using case-based reasoning and genetic algorithms. Journal of Computing in Civil Engineering, 2010. 24(6): p. 499-505.
- Bouabaz, M. and M. Hamami, A cost estimation model for repair bridges based on artificial neural network. American Journal of Applied Sciences, 2008. 5(4): p. 334-339.
- Marzouk, M. and M. Elkadi, Estimating water treatment plants costs using factor analysis and artificial neural networks. Journal of Cleaner Production, 2016. 112: p. 4540-4549.
- Ahiaga-Dagbui, D.D. and S.D. Smith, Neural networks for modelling the final target cost of water projects. 2012.
- Alex, D.P., et al., Artificial neural network model for cost estimation: City of Edmonton’s water and sewer installation services. Journal of construction engineering and management, 2010. 136(7): p. 745-756.
- Petroutsatou, K., et al., Early cost estimating of road tunnel construction using neural networks. Journal of construction engineering and management, 2012. 138(6): p. 679-687.
- Petroutsatou, K. and S. Lambropoulos, Road tunnels construction cost estimation: a structural equation model development and comparison. Operational Research, 2010. 10(2): p. 163-173.
- Gunduz, M., L.O. Ugur, and E. Ozturk, Parametric cost estimation system for light rail transit and metro trackworks. Expert Systems with Applications, 2011. 38(3): p. 2873-2877.
- Kim, B.S., The approximate cost estimating model for railway bridge project in the planning phase using CBR method. KSCE Journal of Civil Engineering, 2011. 15(7): p. 1149.
- Singal, S., R. Saini, and C. Raghuvanshi, Analysis for cost estimation of low head run-of-river small hydropower schemes. Energy for sustainable Development, 2010. 14(2): p. 117-126.
- Gunduz, M. and H.B. Sahin, An early cost estimation model for hydroelectric power plant projects using neural networks and multiple regression analysis. Journal of Civil Engineering and Management, 2015. 21(4): p. 470-477.
- Hashemi, S.T. and H. Kaur, A hybrid conceptual cost estimating model using ANN and GA for power plant projects. Neural Computing and Applications, 2019. 31(7): p. 2143-2154.
- El-Sawalhi, N.I., Modelling the parametric construction project cost estimate using fuzzy logic. International Journal of Emerging Technology and Advanced Engineering Website:(ISSN 2250-2459, Volume 2, Issue 4, 2012. 2(4).
- El-Sawalhi, N.I. and O. Shehatto, A neural network model for building construction projects cost estimating. Journal of Construction Engineering and Project Management, 2014. 4(4): p. 9-16.
- Roxas, C.L.C. and J.M.C. Ongpeng. An artificial neural network approach to structural cost estimation of building projects in the Philippines. in Proc. DLSU Research Congress. 2014.
- Jafarzadeh, R., et al., Application of artificial neural network methodology for predicting seismic retrofit construction costs. Journal of Construction Engineering and Management, 2014. 140(2): p. 04013044.
- Wang, Y.-R., C.-Y. Yu, and H.-H. Chan, Predicting construction cost and schedule success using artificial neural networks ensemble and support vector machines classification models. International Journal of Project Management, 2012. 30(4): p. 470-478.
- Feng, G.L. and L. Li. Application of genetic algorithm and neural network in construction cost estimate. in Advanced materials research. 2013. Trans Tech Publ.
- Kim, S. and J.H. Shim, Combining case-based reasoning with genetic algorithm optimization for preliminary cost estimation in construction industry. Canadian Journal of Civil Engineering, 2014. 41(1): p. 65-73.
- Son, H., C. Kim, and C. Kim, Hybrid principal component analysis and support vector machine model for predicting the cost performance of commercial building projects using pre-project planning variables. Automation in Construction, 2012. 27: p. 60-66.
- Kim, H., L. Soibelman, and F. Grobler, Factor selection for delay analysis using knowledge discovery in databases. Automation in Construction, 2008. 17(5): p. 550-560.
- Mocanu, E., et al., Deep learning for estimating building energy consumption. Sustainable Energy, Grids and Networks, 2016. 6: p. 91-99.
- Rahman, A., V. Srikumar, and A.D. Smith, Predicting electricity consumption for commercial and residential buildings using deep recurrent neural networks. Applied energy, 2018. 212: p. 372-385.
- Rahman, A. and A.D. Smith, Predicting heating demand and sizing a stratified thermal storage tank using deep learning algorithms. Applied Energy, 2018. 228: p. 108-121.
- Olawumi, T.O. and D.W. Chan, A scientometric review of global research on sustainability and sustainable development. Journal of cleaner production, 2018. 183: p. 231-250.
- Montoya, F.G., et al., The research on energy in Spain: A scientometric approach. Renewable and Sustainable Energy Reviews, 2014. 29: p. 173-183.
- Darko, A., et al., A scientometric analysis and visualization of global green building research. Building and Environment, 2019. 149: p. 501-511.
- Xiao, X., et al., Mapping knowledge in the economic areas of green building using scientometric analysis. Energies, 2019. 12(15): p. 3011.
- Wu, Z., et al., A scientometric review of system dynamics applications in construction management research. Sustainability, 2020. 12(18): p. 7474.
- Liu, Z., Y. Lu, and L.C. Peh, A review and scientometric analysis of global building information modeling (BIM) research in the architecture, engineering and construction (AEC) industry. Buildings, 2019. 9(10): p. 210.
- Van Tam, N., Scientometric Review of Research Trends on Public Private Partnership (PPP) for Infrastructure Projects From 2000 to 2020. 2021. Vol. 4. 2021. https://doi.org/10.32783/csid-jid.v4i1.215
- de Toledo, R.F., et al., A scientometric review of global research on sustainability and project management dataset. Data in brief, 2019. 25: p. 104312.
- Zhao, X., et al., A bibliometric review of green building research 2000–2016. Architectural Science Review, 2019. 62(1): p. 74-88.
- Meho, L.I. and Y. Rogers, Citation counting, citation ranking, and h-index of human-computer interaction researchers: a comparison of Scopus and Web of Science. Journal of the American Society for Information Science and Technology, 2008. 59(11): p. 1711-1726.
- Li, H., J.N. Cao, and P.E.D. Love, Using machine learning and GA to solve time-cost trade-off problems. Journal of Construction Engineering and Management, 1999. 125(5): p. 347-353.
- Al-Tabtabai, H. and A.P. Alex, Using genetic algorithms to solve optimization problems in construction. Engineering, Construction and Architectural Management, 1999. 6(2): p. 121-132.
- Shrivastava, R. and P. Mahajan, Artificial intelligence research in India: A scientometric analysis. Science & Technology Libraries, 2016. 35(2): p. 136-151.
- Wuni, I.Y., G.Q. Shen, and R. Osei-Kyei, Scientometric review of global research trends on green buildings in construction journals from 1992 to 2018. Energy and buildings, 2019. 190: p. 69-85.
- Van Eck, N.J. and L. Waltman, Visualizing bibliometric networks, in Measuring scholarly impact. 2014, Springer. p. 285-320.
- Van Eck, N. and L. Waltman, Manual for VOSviewer version 1.6. 8. CWTS Meaningful Metrics. Universiteit Leiden, 2018.
- Akinosho, T.D., et al., Deep learning in the construction industry: A review of present status and future innovations. Journal of Building Engineering, 2020. 32.