Skin Lesion Classification Based on Convolutional Neural Network
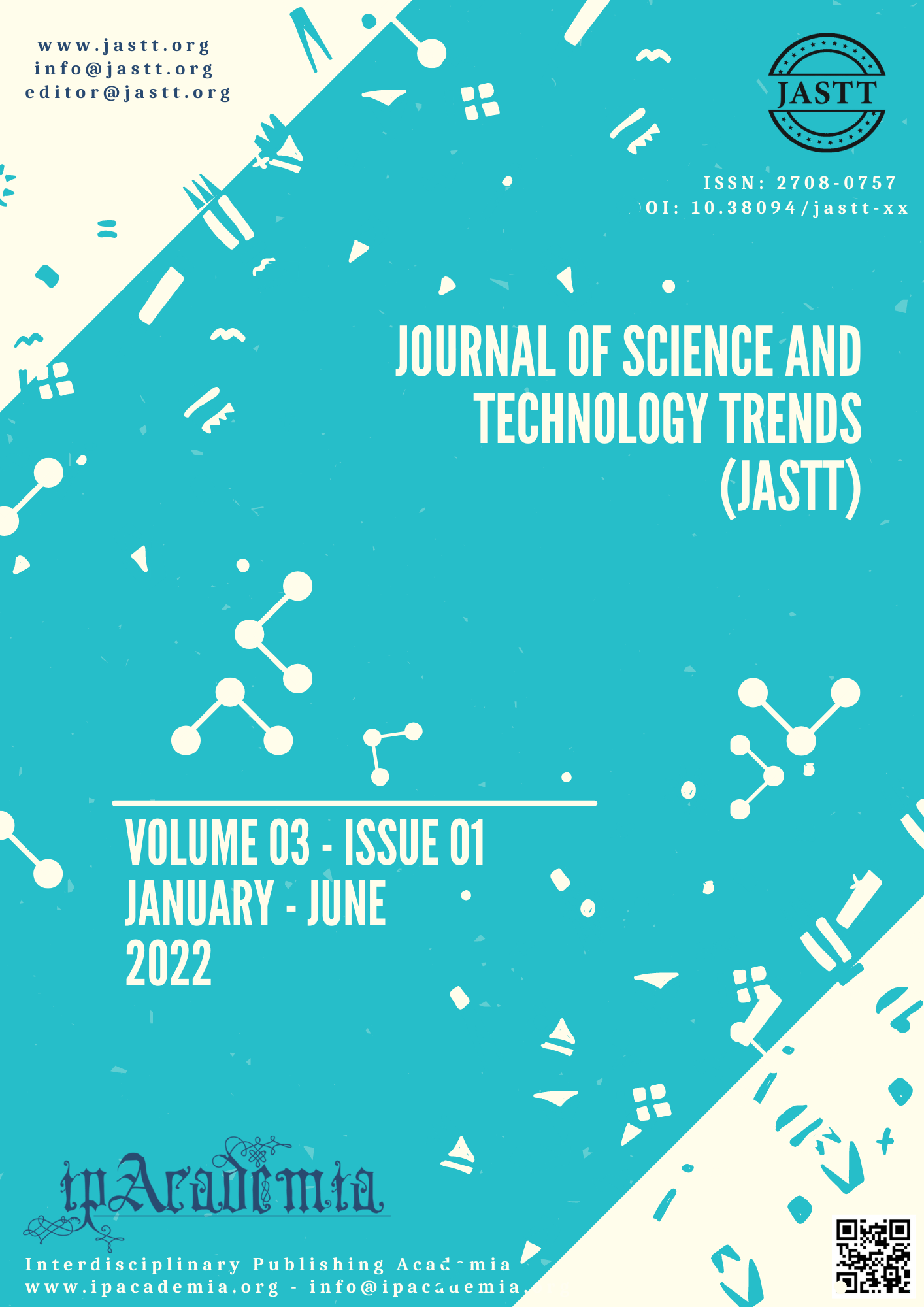
Abstract
Skin cancer is one of the most common cancers, and its early detection can have a huge impact on its outcomes. Deep learning, especially convolutional neural networks, perform well in processing massive amounts of data, especially image data in classifying skin cancer. In this paper, convolutional neural networks are mainly used to diagnose and classify 7 types of skin lesions, including melanoma, basal cell carcinoma, melanocytic nevus, actinic keratosis, and intraepithelial carcinoma, benign keratinoid lesions, dermatofibroma, and vascular lesions. First, the characteristics of skin lesion images are analyzed, using image processing technology and sampling technology to preprocess skin lesion images. Then the training parameters of imageNet network are adjusted through the idea of transfer learning on InceptionV3, ResNet50, DenseNet201, and other networks to perform training classification. Furthermore, different convolutional neural network models are built for classification. In order to validate the classification performance of various convolutional neural network models, this paper adopts ISIC 2017 HAM10000 dataset for experiments. The experimental results show that proper preprocessing is necessary when applying CNN for image classification. In classifying the 224*224 skin lesion images, the classical deep convolutional network with DenseNet201 achieved a remarkable performance classification accuracy of 99.12% for training and 86.91% for testing.
Keywords
Convolutional Neural Networks, Image Processing, Skin Cancer, Skin Lesion Images, Deep Convolutional Neural Network
References
- P. J. Limburg, D. A. Ahlquist, S. Johnson, S. Jayasekar Zurn, J. B. Kisiel, and R. A. Smith, “Multicancer early detection: International summit to Clarify the Roadmap,” Cancer, vol. 128, pp. 859–860, 2022.
- C. R. Pickering et al., “Mutational Landscape of Aggressive Cutaneous Squamous Cell Carcinoma,” Clin Cancer Res, vol. 20, no. 24, pp. 6582–6592, Dec. 2014, doi: 10.1158/1078-0432.CCR-14-1768.
- J. G. Muzic et al., “Incidence and Trends of Basal Cell Carcinoma and Cutaneous Squamous Cell Carcinoma: A Population-Based Study in Olmsted County, Minnesota, 2000 to 2010,” Mayo Clinic Proceedings, vol. 92, no. 6, pp. 890–898, Jun. 2017, doi: 10.1016/j.mayocp.2017.02.015.
- M. n. Manahan et al., “A pilot trial of mobile, patient-performed teledermoscopy,” British Journal of Dermatology, vol. 172, no. 4, pp. 1072–1080, 2015, doi: 10.1111/bjd.13550.
- K. Clebak, “Dermatoscopy and Skin Cancer: A Handbook for Hunters of Skin Cancer and Melanoma,” Family Medicine, vol. 52, no. 2, pp. 148–149, 2020, doi: 10.22454/FamMed.2020.191543.
- H. G. Demirdag and B. Tugrul, “Evaluation of relationship between antihypertensive drug usage and dermatoscopic features in patients with keratinizing skin cancer,” Dermatologic Therapy, vol. 34, no. 4, p. e14957, 2021, doi: 10.1111/dth.14957.
- S. S. Han et al., “Keratinocytic Skin Cancer Detection on the Face Using Region-Based Convolutional Neural Network,” JAMA Dermatology, vol. 156, no. 1, pp. 29–37, Jan. 2020, doi: 10.1001/jamadermatol.2019.3807.
- “Development and Validation of a Deep Learning Algorithm for Detection of Diabetic Retinopathy in Retinal Fundus Photographs | Diabetic Retinopathy | JAMA | JAMA Network.” https://jamanetwork.com/journals/jama/fullarticle/2588763/ (accessed Apr. 14, 2022).
- “Deep-learning-based, computer-aided classifier developed with a small dataset of clinical images surpasses board-certified dermatologists in skin tumour diagnosis - Fujisawa - 2019 - British Journal of Dermatology - Wiley Online Library.” https://onlinelibrary.wiley.com/doi/full/10.1111/bjd.16924?casa_token=dDWfx0W_eeoAAAAA%3AJlWu29mzLZBk7k0m7BIg1vaCn1kOzIrLGYmc7IjzBfAXo9_VwF0MDIFkcriADWyd9Z0Lve-_VmbAJizrNQ (accessed Apr. 14, 2022).
- “Expert-Level Diagnosis of Nonpigmented Skin Cancer by Combined Convolutional Neural Networks | Dermatology | JAMA Dermatology | JAMA Network.” https://jamanetwork.com/journals/jamadermatology/article-abstract/2716294 (accessed Apr. 14, 2022).
- R. C. Maron et al., “Systematic outperformance of 112 dermatologists in multiclass skin cancer image classification by convolutional neural networks,” European Journal of Cancer, vol. 119, pp. 57–65, Sep. 2019, doi: 10.1016/j.ejca.2019.06.013.
- F. P. Such, V. Madhavan, E. Conti, J. Lehman, K. O. Stanley, and J. Clune, “Deep Neuroevolution: Genetic Algorithms Are a Competitive Alternative for Training Deep Neural Networks for Reinforcement Learning,” arXiv:1712.06567 [cs], Apr. 2018, Accessed: Apr. 14, 2022. [Online]. Available: http://arxiv.org/abs/1712.06567
- Y. Veisani, E. Jenabi, S. Khazaei, and Sh. Nematollahi, “Global incidence and mortality rates in pancreatic cancer and the association with the Human Development Index: decomposition approach,” Public Health, vol. 156, pp. 87–91, Mar. 2018, doi: 10.1016/j.puhe.2017.12.015.
- N. M. Alwani, S. Fatima, B. K. Adiga, and N. Haider, “Primary melanoma of cecum: A diagnostic challenge,” Indian Journal of Pathology and Microbiology, vol. 62, no. 4, p. 641, 2019.
- C. Qian et al., “A detection and segmentation architecture for skin lesion segmentation on dermoscopy images,” arXiv preprint arXiv:1809.03917, 2018.
- F. Erçal, H.-C. Lee, W. V. Stoecker, and R. H. Moss, “Skin cancer diagnosis using hierarchical neural networks and fuzzy systems,” 1994.
- R. Mishra, Computer Aided Diagnosis Systems for Digital Analysis of Osteosarcoma and Skin Cancer. The University of Texas at Dallas, 2019.
- M. Binder, A. Steiner, M. Schwarz, S. Knollmayer, K. Wolff, and H. Pehamberger, “Application of an artificial neural network in epiluminescence microscopy pattern analysis of pigmented skin lesions: a pilot study,” British Journal of Dermatology, vol. 130, no. 4, pp. 460–465, 1994.
- A. Mahbod, G. Schaefer, I. Ellinger, R. Ecker, A. Pitiot, and C. Wang, “Fusing fine-tuned deep features for skin lesion classification,” Computerized Medical Imaging and Graphics, vol. 71, pp. 19–29, Jan. 2019, doi: 10.1016/j.compmedimag.2018.10.007.
- J. Kawahara, A. BenTaieb, and G. Hamarneh, “Deep features to classify skin lesions,” in 2016 IEEE 13th international symposium on biomedical imaging (ISBI), 2016, pp. 1397–1400.
- J. C. Kavitha and A. Suruliandi, “Texture and color feature extraction for classification of melanoma using SVM,” in 2016 International conference on computing technologies and intelligent data engineering (ICCTIDE’16), 2016, pp. 1–6.
- J. Zhang, Y. Xie, Y. Xia, and C. Shen, “Attention residual learning for skin lesion classification,” IEEE transactions on medical imaging, vol. 38, no. 9, pp. 2092–2103, 2019.
- L. Bi, D. D. Feng, M. Fulham, and J. Kim, “Multi-label classification of multi-modality skin lesion via hyper-connected convolutional neural network,” Pattern Recognition, vol. 107, p. 107502, 2020.
- J. Bernat García, M. M. Morales Suárez-Varela, J. J. Vilata Corell, A. Marquina Vila, L. M. Pallardó Mateu, and J. Crespo, “Risk factors for non-melanoma skin cancer in kidney transplant patients in a Spanish population in the Mediterranean region,” Bernat García, Josefa; Morales Suárez-Varela, María; Vilata, Juan José; Marquina Vila, Amparo; Pallardó, Luis; Crespo, Josep (2013) Risk factors for non-melanoma skin cancer in kidney transplant patients in a Spanish population in the Mediterranean region Acta Dermato-Venereologica 4 93 422 427, 2013, doi: 10.2340/00015555-1525.
- D. B. Mendes and N. C. da Silva, “Skin Lesions Classification Using Convolutional Neural Networks in Clinical Images,” arXiv:1812.02316 [cs], Dec. 2018, Accessed: Apr. 14, 2022. [Online]. Available: http://arxiv.org/abs/1812.02316
- Z. Gao et al., “Learning physical properties in complex visual scenes: An intelligent machine for perceiving blood flow dynamics from static CT angiography imaging,” Neural Networks, vol. 123, pp. 82–93, Mar. 2020, doi: 10.1016/j.neunet.2019.11.017.