Region of Interest Segmentation Based on Clustering Techniques for Breast Cancer Ultrasound Images: A Review
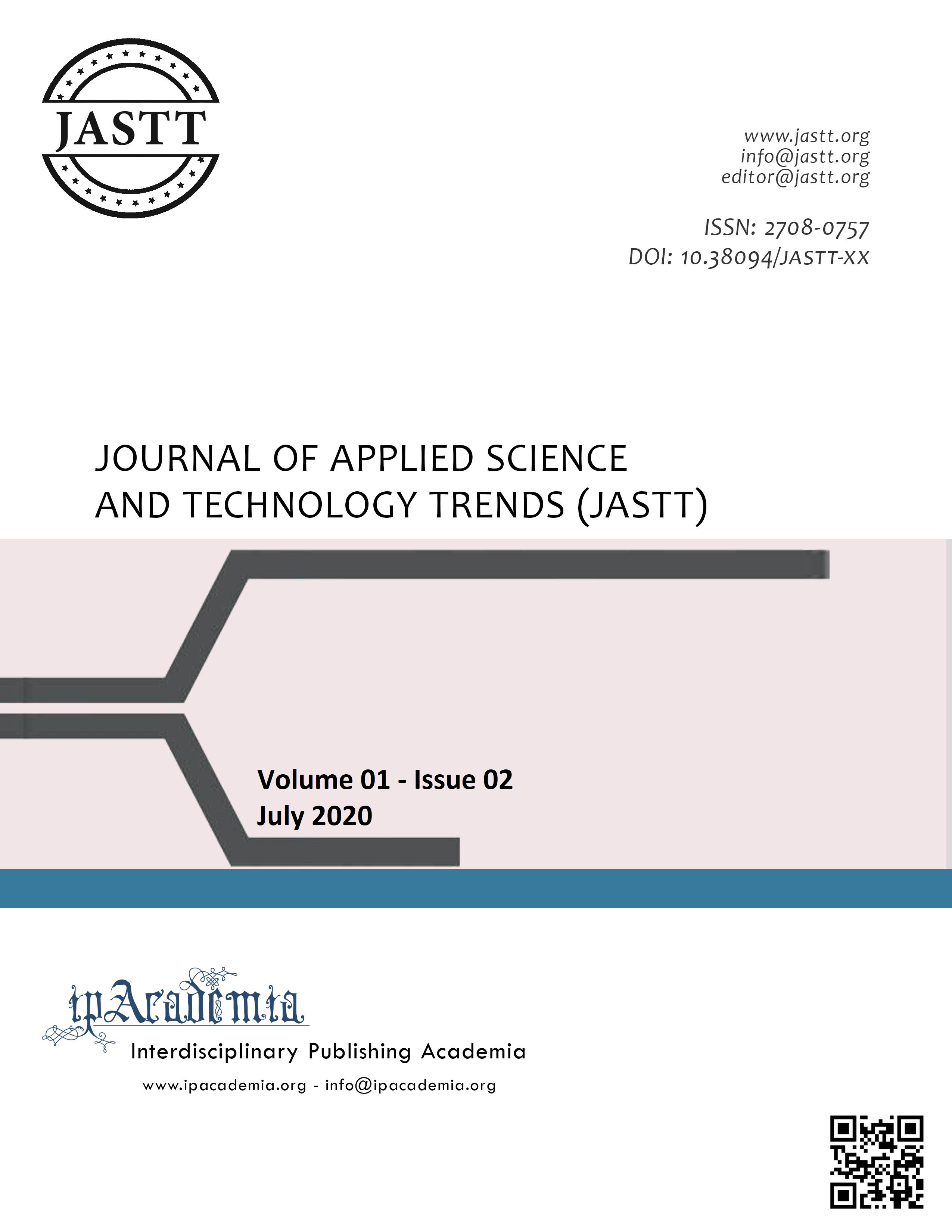
Abstract
The most prevalent cancer amongst women is woman breast cancer. Ultrasound imaging is a widely employed method for identifying and diagnosing breast abnormalities. Computer-aided diagnosis technologies have lately been developed with ultrasound images to help radiologists enhance the accuracy of the diagnosis. This paper presents several ultrasound image segmentation techniques, mainly focus on eight clustering methods over the last 10 years, and it shows the advantages and disadvantages of these approaches. Breast ultrasound image segmentation is, therefore, still an accessible and challenging issue due to numerous ultrasound artifacts introduced in the imaging process, including high speckle noise, poor contrast, blurry edges, weak signal-to-noise ratio, and intensity inhomogeneity.
Keywords
Breast cancer, Computer-aided diagnosis, Ultrasound image, Segmentation, Clustering
References
- D. M. Abdulqader, A. M. Abdulazeez, and D. Q. Zeebaree, ‘Machine Learning Supervised Algorithms of Gene Selection: A Review’, Mach. Learn., vol. 62, no. 03, 2020.
- Q. Huang, Y. Luo, and Q. Zhang, ‘Breast ultrasound image segmentation: a survey’, Int. J. Comput. Assist. Radiol. Surg., vol. 12, no. 3, pp. 493–507, 2017.
- H.-D. Cheng, X. Shi, R. Min, L. Hu, X. Cai, and H. Du, ‘Approaches for automated detection and classification of masses in mammograms’, Pattern Recognit., vol. 39, no. 4, pp. 646–668, 2006.
- H.-D. Cheng, X. Cai, X. Chen, L. Hu, and X. Lou, ‘Computer-aided detection and classification of microcalcifications in mammograms: a survey’, Pattern Recognit., vol. 36, no. 12, pp. 2967–2991, 2003.
- Lee, Carol H. "Screening mammography: proven benefit, continued controversy." Radiologic Clinics of North America 40.3 (2002): 395-407.
- Yaseen, D. S., Batumalai, S. A., Ahmed, F. Y., & Fong, S. L. (2019, August). Improved Disabled Mobile Aid Application for Android: Health and Fitness Helper for Disabled People. In 2019 IEEE 10th Control and System Graduate Research Colloquium (ICSGRC) (pp. 207-212).
- D. Q. Zeebaree, H. Haron, and A. M. Abdulazeez, ‘Gene selection and classification of microarray data using convolutional neural network’, presented at the 2018 International Conference on Advanced Science and Engineering (ICOASE), 2018, pp. 145–150.
- J. L. Jesneck, J. Y. Lo, and J. A. Baker, ‘Breast mass lesions: computer-aided diagnosis models with mammographic and sonographic descriptors’, Radiology, vol. 244, no. 2, pp. 390–398, 2007.
- R. E. Bird, T. W. Wallace, and B. C. Yankaskas, ‘Analysis of cancers missed at screening mammography.’, Radiology, vol. 184, no. 3, pp. 613–617, 1992.
- M. L. Giger, ‘Computer-aided diagnosis in radiology’, Acad. Radiol., vol. 9, no. 1, pp. 1–3, 2002.
- B. Sahiner et al., ‘Malignant and benign breast masses on 3D US volumetric images: effect of computer-aided diagnosis on radiologist accuracy’, Radiology, vol. 242, no. 3, pp. 716–724, 2007.
- Zebari, D. A., Haron, H., Zeebaree, D. Q., & Zain, A. M. (2019, August). A Simultaneous Approach for Compression and Encryption Techniques Using Deoxyribonucleic Acid. In 2019 13th International Conference on Software, Knowledge, Information Management and Applications (SKIMA) (pp. 1-6). IEEE.
- B. O. Anderson et al., ‘Breast cancer in limited resource countries: an overview of the Breast Health Global Initiative 2005 guidelines’, Breast J., vol. 12, pp. S3–S15, 2006.
- P. Shankar, C. Piccoli, J. Reid, F. Forsberg, and B. Goldberg, ‘Application of the compound probability density function for characterization of breast masses in ultrasound B scans’, Phys. Med. Biol., vol. 50, no. 10, p. 2241, 2005.
- K. Drukker, M. Giger, L. A. Meinel, A. Starkey, J. Janardanan, and H. Abe, ‘Quantitative ultrasound image analysis of axillary lymph node status in breast cancer patients’, Int. J. Comput. Assist. Radiol. Surg., vol. 8, no. 6, pp. 895–903, 2013.
- R.-F. Chang, W.-J. Wu, W. K. Moon, and D.-R. Chen, ‘Improvement in breast tumor discrimination by support vector machines and speckle-emphasis texture analysis’, Ultrasound Med. Biol., vol. 29, no. 5, pp. 679–686, 2003.
- C.-M. Chen et al., ‘Breast lesions on sonograms: computer-aided diagnosis with nearly setting-independent features and artificial neural networks’, Radiology, vol. 226, no. 2, pp. 504–514, 2003.
- M. P. André, M. Galperin, L. K. Olson, K. Richman, S. Payrovi, and P. Phan, ‘Improving the accuracy of diagnostic breast ultrasound’, in Acoustical Imaging, Springer, 2002, pp. 453–460.
- F. G. Y. Cikla Candir, A. Ertaylan, U. Binzat, and A. Kut, ‘Lesion Detection from the Ultrasound Images Using K-Means Algorithm’, presented at the 2019 Medical Technologies Congress (TIPTEKNO), 2019, pp. 1–4.
- L. Zhang, Y. Ren, C. Huang, and F. Liu, ‘A novel automatic tumor detection for breast cancer ultrasound images’, presented at the 2011 Eighth International Conference on Fuzzy Systems and Knowledge Discovery (FSKD), 2011, vol. 1, pp. 401–404.
- K. S. Camilus and V. Govindan, ‘A Review on Graph Based Segmentation.’, Int. J. Image Graph. Signal Process., vol. 4, no. 5, 2012.
- C.-C. Kang, W.-J. Wang, and C.-H. Kang, ‘Image segmentation with complicated background by using seeded region growing’, AEU-Int. J. Electron. Commun., vol. 66, no. 9, pp. 767–771, 2012.
- K. Prabusankarlal, P. Thirumoorthy, and R. Manavalan, ‘Computer aided breast cancer diagnosis techniques in ultrasound: a survey’, J. Med. Imaging Health Inform., vol. 4, no. 3, pp. 331–349, 2014.
- Mazaheri, S., Sulaiman, P. S. B., Wirza, R., Khalid, F., Kadiman, S., Dimon, M. Z., & Tayebi, R. M. (2013, December). Echocardiography image segmentation: A survey. In 2013 International Conference on Advanced Computer Science Applications and Technologies (pp. 327-332). IEEE.
- P. S. Rodrigues and G. A. Giraldi, ‘Improving the non-extensive medical image segmentation based on Tsallis entropy’, Pattern Anal. Appl., vol. 14, no. 4, pp. 369–379, 2011.s
- Huang, Yu-Len, Kao-Lun Wang, and Dar-Ren Chen. "Diagnosis of breast tumors with ultrasonic texture analysis using support vector machines." Neural Computing & Applications 15.2 (2006): 164-169.
- D. Q. Zeebaree, H. Haron, A. M. Abdulazeez, and D. A. Zebari, ‘Machine learning and Region Growing for Breast Cancer Segmentation’, presented at the 2019 International Conference on Advanced Science and Engineering (ICOASE), 2019, pp. 88–93.
- M. Xian et al., A benchmark for breast ultrasound image segmentation (BUSIS). Infinite Study, 2018. https://arxiv.org/ftp/arxiv/papers/1801/1801.03182.pdf
- M. Lal, L. Kaur, and S. Gupta, ‘Modified spatial neutrosophic clustering technique for boundary extraction of tumours in B-mode BUS images’, IET Image Process., vol. 12, no. 8, pp. 1338–1344, 2018.
- A. Ibrahim, S. Mohammed, and H. A. Ali, ‘Breast cancer detection and classification using thermography: a review’, presented at the International Conference on Advanced Machine Learning Technologies and Applications, 2018, pp. 496–505.
- R. Zebari, A. Abdulazeez, D. Zeebaree, D. Zebari, and J. Saeed, ‘A Comprehensive Review of Dimensionality Reduction Techniques for Feature Selection and Feature Extraction’, J. Appl. Sci. Technol. Trends, vol. 1, no. 2, pp. 56–70, 2020.
- O. Ahmed and A. Brifcani, ‘Gene Expression Classification Based on Deep Learning’, presented at the 2019 4th Scientific International Conference Najaf (SICN), 2019, pp. 145–149.
- J. N. Saeed, ‘A SURVEY OF ULTRASONOGRAPHY BREAST CANCER IMAGE SEGMENTATION TECHNIQUES’, Acad. J. Nawroz Univ., vol. 9, no. 1, pp. 1–14, 2020.
- D. Q. Zeebaree, H. Haron, A. M. Abdulazeez, and S. R. Zeebaree, ‘Combination of K-means clustering with Genetic Algorithm: A review’, Int. J. Appl. Eng. Res., vol. 12, no. 24, pp. 14238–14245, 2017.
- Ahmed, F. Y., Shamsuddin, S. M., & Hashim, S. Z. M. (2013). Improved SpikeProp for using particle swarm optimization. Mathematical Problems in Engineering, 2013., V 2013, http://dx.doi.org/10.1155/2013/257085.
- N. Adeen, M. Abdulazeez, and D. Zeebaree, ‘Systematic Review of Unsupervised Genomic Clustering Algorithms Techniques for High Dimensional Datasets’, vol. 62, no. 3, 2020.
- Najat, N., & Abdulazeez, A. M. (2017, November). Gene clustering with partition around mediods algorithm based on weighted and normalized Mahalanobis distance. In 2017 International Conference on Intelligent Informatics and Biomedical Sciences (ICIIBMS) (pp. 140-145). IEEE.
- National Research Council, Mammography and beyond: developing technologies for the early detection of breast cancer. National Academies Press, 2001.Book
- P. Thompson, ‘Ultrasound in Obstetrics and Gynaecology: European Practice in Gynaecology and Obstetrics’, 2010. 30(8), pp. 887–888.
- K. Johnson, D. Sarma, and E. S. Hwang, ‘Lobular breast cancer series: imaging’, Breast Cancer Res., vol. 17, no. 1, p. 94, 2015.
- Adeen, I. M. N., Abdulazeez, A. M., & Zeebaree, D. Q. Systematic Review of Unsupervised Genomic Clustering Algorithms Techniques for High Dimensional Datasets., vol. 62, no. 03, 2020.
- K. Drukker, M. L. Giger, K. Horsch, M. A. Kupinski, C. J. Vyborny, and E. B. Mendelson, ‘Computerized lesion detection on breast ultrasound’, Med. Phys., vol. 29, no. 7, pp. 1438–1446, 2002.
- K. Horsch, M. L. Giger, L. A. Venta, and C. J. Vyborny, ‘Automatic segmentation of breast lesions on ultrasound’, Med. Phys., vol. 28, no. 8, pp. 1652–1659, 2001.
- K. Prabusankarlal, P. Thirumoorthy, and R. Manavalan, ‘Segmentation of breast lesions in ultrasound images through multiresolution analysis using undecimated discrete wavelet transform’, Ultrason. Imaging, vol. 38, no. 6, pp. 384–402, 2016.
- D. A. Zebari, H. Haron, S. R. Zeebaree, and D. Q. Zeebaree, ‘Enhance the Mammogram Images for Both Segmentation and Feature Extraction Using Wavelet Transform’, presented at the 2019 International Conference on Advanced Science and Engineering (ICOASE), 2019, pp. 100–105.
- E. Samundeeswari, P. Saranya, and R. Manavalan, ‘Segmentation of breast ultrasound image using regularized K-means (ReKM) clustering’, presented at the 2016 International Conference on Wireless Communications, Signal Processing and Networking (WiSPNET), 2016, pp. 1379–1383.
- Y.-L. Huang and D.-R. Chen, ‘Watershed segmentation for breast tumor in 2-D sonography’, Ultrasound Med. Biol., vol. 30, no. 5, pp. 625–632, 2004.
- C.-M. Lo et al., ‘Multi-dimensional tumor detection in automated whole breast ultrasound using topographic watershed’, IEEE Trans. Med. Imaging, vol. 33, no. 7, pp. 1503–1511, 2014.
- H. A. Nugroho, Y. Triyani, M. Rahmawaty, and I. Ardiyanto, ‘Breast ultrasound image segmentation based on neutrosophic set and watershed method for classifying margin characteristics’, presented at the 2017 7th IEEE International Conference on System Engineering and Technology (ICSET), 2017, pp. 43–47.
- H. A. Nugroho, Y. Triyani, M. Rahmawaty, and I. Ardiyanto, ‘Analysis of margin sharpness for breast nodule classification on ultrasound images’, presented at the 2017 9th International Conference on Information Technology and Electrical Engineering (ICITEE), 2017, pp. 1–5.
- Q.-H. Huang, S.-Y. Lee, L.-Z. Liu, M.-H. Lu, L.-W. Jin, and A.-H. Li, ‘A robust graph-based segmentation method for breast tumors in ultrasound images’, Ultrasonics, vol. 52, no. 2, pp. 266–275, 2012.
- N. M. A. Ahmed and A. M. A. Brifcani, ‘A new modified embedded zerotree wavelet approach for image coding (nmezw)’, Int. J. Sci. Eng. Res., vol. 4, 2013.
- Y.-L. Huang, Y.-R. Jiang, D.-R. Chen, and W. K. Moon, ‘Level set contouring for breast tumor in sonography’, J. Digit. Imaging, vol. 20, no. 3, pp. 238–247, 2007.
- R. V. Menon, P. Raha, S. Kothari, S. Chakraborty, I. Chakrabarti, and R. Karim, ‘Automated detection and classification of mass from breast ultrasound images’, presented at the 2015 Fifth National Conference on Computer Vision, Pattern Recognition, Image Processing and Graphics (NCVPRIPG), 2015, pp. 1–4.
- W. Khan, ‘Image segmentation techniques: A survey’, J. Image Graph., vol. 1, no. 4, pp. 166–170, 2013.
- X. Jiang, Y. Guo, H. Chen, Y. Zhang, and Y. Lu, ‘An adaptive region growing based on neutrosophic set in ultrasound domain for image segmentation’, IEEE Access, vol. 7, pp. 60584–60593, 2019.
- A. Takemura, A. Shimizu, and K. Hamamoto, ‘A cost-sensitive extension of AdaBoost with markov random field priors for automated segmentation of breast tumors in ultrasonic images’, Int. J. Comput. Assist. Radiol. Surg., vol. 5, no. 5, pp. 537–547, 2010.
- M. Xian, J. Huang, Y. Zhang, and X. Tang, ‘Multiple-domain knowledge based MRF model for tumor segmentation in breast ultrasound images’, presented at the 2012 19th IEEE International Conference on Image Processing, 2012, pp. 2021–2024.
- P. F. Felzenszwalb and D. P. Huttenlocher, ‘Efficient graph-based image segmentation’, Int. J. Comput. Vis., vol. 59, no. 2, pp. 167–181, 2004.
- A. Vedaldi and S. Soatto, ‘Quick shift and kernel methods for mode seeking’, presented at the European conference on computer vision, 2008, pp. 705–718.
- R. Achanta, A. Shaji, K. Smith, A. Lucchi, P. Fua, and S. Süsstrunk, ‘SLIC superpixels compared to state-of-the-art superpixel methods’, IEEE Trans. Pattern Anal. Mach. Intell., vol. 34, no. 11, pp. 2274–2282, 2012.
- J.-S. Lee, ‘Digital image smoothing and the sigma filter’, Comput. Vis. Graph. Image Process., vol. 24, no. 2, pp. 255–269, 1983.
- N. N. Mohammed and A. M. Abdulazeez, ‘Evaluation of partitioning around medoids algorithm with various distances on microarray data’, presented at the 2017 IEEE International Conference on Internet of Things (iThings) and IEEE Green Computing and Communications (GreenCom) and IEEE Cyber, Physical and Social Computing (CPSCom) and IEEE Smart Data (SmartData), 2017, pp. 1011–1016.
- Kekre, H. B., and Pravin Shrinath. "Tumour Delineation using Statistical Properties of The Breast US Images and Vector Quantization based Clustering Algorithms." International Journal of Image, Graphics & Signal Processing 5.11 (2013).
- Z. Fang, W. Zhang, and H. Ma, ‘Breast Cancer Classification with Ultrasound Images Based on SLIC’, ArXiv Prepr. ArXiv190411322, 2019.
- A. Al-Zebari and A. Sengur, ‘Performance Comparison of Machine Learning Techniques on Diabetes Disease Detection’, presented at the 2019 1st International Informatics and Software Engineering Conference (UBMYK), 2019, pp. 1–4.
- S. S. Khan and A. Ahmad, ‘Cluster center initialization algorithm for K-means clustering’, Pattern Recognit. Lett., vol. 25, no. 11, pp. 1293–1302, 2004.
- E. Samundeeswari, P. Saranya, and R. Manavalan, ‘M2 Filter For Speckle Noise Suppression In Breast Ultrasound Images’, ICTACT J. Image Video Process., vol. 6, no. 2, pp. 1137–1144, 2015.
- J. Guo, E. Levina, G. Michailidis, and J. Zhu, ‘Pairwise variable selection for high dimensional model based clustering’, Biometrics, vol. 66, no. 3, pp. 793–804, 2010.
- D. M. Sulaiman, A. M. Abdulazeez, H. Haron, and S. S. Sadiq, ‘Unsupervised Learning Approach-Based New Optimization K-Means Clustering for Finger Vein Image Localization’, presented at the 2019 International Conference on Advanced Science and Engineering (ICOASE), 2019, pp. 82–87.
- J. C. Dunn, ‘A fuzzy relative of the ISODATA process and its use in detecting compact well-separated clusters’, V3-1973, issue 3.
- J. C. Bezdek, Pattern recognition with fuzzy objective function algorithms. Springer Science & Business Media, 2013, Book.
- J. Miao, X. Zhou, and T.-Z. Huang, ‘Local segmentation of images using an improved fuzzy C-means clustering algorithm based on self-adaptive dictionary learning’, Appl. Soft Comput., p. 106200, 2020.
- L. Panigrahi, K. Verma, and B. K. Singh, ‘Ultrasound image segmentation using a novel multi-scale Gaussian kernel fuzzy clustering and multi-scale vector field convolution’, Expert Syst. Appl., vol. 115, pp. 486–498, 2019.
- N. Alamelumangai and J. Devishree, ‘Automated clustering of cancer cells using fuzzy C means with repulsions in ultrasound images’, J. Artif. Intell., vol. 5, pp. 14–25, 2012.
- D. Naik and P. Shah, ‘A review on image segmentation clustering algorithms’, Int J Comput Sci Inf. Technol, vol. 5, no. 3, pp. 3289–93, 2014.
- Zeebaree, D. Q., Haron, H., Abdulazeez, A. M., & Zebari, D. A. (2019, April). Trainable Model Based on New Uniform LBP Feature to Identify the Risk of the Breast Cancer. In 2019 International Conference on Advanced Science and Engineering (ICOASE) (pp. 106-111). IEEE.
- S. Tatiraju and A. Mehta, ‘Image Segmentation using k-means clustering, EM and Normalized Cuts’, Dep. EECS, vol. 1, pp. 1–7, 2008.
- K. Y. Yeung, C. Fraley, A. Murua, A. E. Raftery, and W. L. Ruzzo, ‘Model-based clustering and data transformations for gene expression data’, Bioinformatics, vol. 17, no. 10, pp. 977–987, 2001.
- P. Lukac, R. Hudec, M. Benco, P. Kamencay, Z. Dubcova, and M. Zachariasova, ‘Simple comparison of image segmentation algorithms based on evaluation criterion’, presented at the Proceedings of 21st International Conference Radioelektronika 2011, 2011, pp. 1–4.
- E. R. Dougherty et al., ‘Inference from clustering with application to gene-expression microarrays’, J. Comput. Biol., vol. 9, no. 1, pp. 105–126, 2002.
- D. Comaniciu, V. Ramesh, and P. Meer, ‘The variable bandwidth mean shift and data-driven scale selection’, presented at the Proceedings Eighth IEEE International Conference on Computer Vision. ICCV 2001, 2001, vol. 1, pp. 438–445.
- K. Fukunaga and L. Hostetler, ‘The estimation of the gradient of a density function, with applications in pattern recognition’, IEEE Trans. Inf. Theory, vol. 21, no. 1, pp. 32–40, 1975.
- Y. Cheng, ‘Mean shift, mode seeking, and clustering’, IEEE Trans. Pattern Anal. Mach. Intell., vol. 17, no. 8, pp. 790–799, 1995.
- D. Comaniciu and P. Meer, ‘Mean shift: A robust approach toward feature space analysis’, IEEE Trans. Pattern Anal. Mach. Intell., vol. 24, no. 5, pp. 603–619, 2002.
- J. Carballido-Gamio, S. J. Belongie, and S. Majumdar, ‘Normalized cuts in 3-D for spinal MRI segmentation’, IEEE Trans. Med. Imaging, vol. 23, no. 1, pp. 36–44, 2004.
- A. Rosenfeld, ‘Fuzzy digital topology’, Inf. Control, vol. 40, no. 1, pp. 76–87, 1979.
- L. Chen and J. Zhang, ‘Fuzzy subfiber and its application to seismic lithology classification’, Inf. Sci.-Appl., vol. 1, no. 2, pp. 77–95, 1994.
- M. Xian, H.-D. Cheng, and Y. Zhang, ‘A fully automatic breast ultrasound image segmentation approach based on neutro-connectedness’, presented at the 2014 22nd International Conference on Pattern Recognition, 2014, pp. 2495–2500.
- O. M. S. Hassan, A. M. Abdulazeez, and V. M. TIRYAKI, ‘Gait-based human gender classification using lifting 5/3 wavelet and principal component analysis’, presented at the 2018 International Conference on Advanced Science and Engineering (ICOASE), 2018, pp. 173–178.
- Norouzi, A., Rahim, M. S. M., Altameem, A., Saba, T., Rad, A. E., Rehman, A., & Uddin, M. (2014). Medical image segmentation methods, algorithms, and applications. IETE Technical Review, 31(3), 199-213.
- Xian, M., Zhang, Y., Cheng, H. D., Xu, F., Zhang, B., & Ding, J. (2018). Automatic breast ultrasound image segmentation: A survey. Pattern Recognition, 79, 340-355.
- Feng, W., Xiang, H., & Zhu, Y. (2011, May). An improved graph-based image segmentation algorithm and its gpu acceleration. In 2011 Workshop on Digital Media and Digital Content Management (pp. 237-241). IEEE.
- Cigla, C., & Alatan, A. A. (2010, September). Efficient graph-based image segmentation via speeded-up turbo pixels. In 2010 IEEE International Conference on Image Processing (pp. 3013-3016). IEEE.
- Sharma, P., & Suji, J. (2016). A review on image segmentation with its clustering techniques. International Journal of Signal Processing, Image Processing and Pattern Recognition, 9(5), 209-218.
- Qin, F., Guo, J., & Lang, F. (2014). Superpixel segmentation for polarimetric SAR imagery using local iterative clustering. IEEE Geoscience and Remote Sensing Letters, 12(1), 13-17.
- Grover, N. (2014). A study of various fuzzy clustering algorithms. International Journal of Engineering Research, 3(3), 177-181.
- Tolba, M. F., Mostafa, M. G., Gharib, T. F., & Salem, M. A. M. (2003). MR-Brain Image Segmentation Using Gaussian Multiresolution Analysis and the EM Algorithm. In ICEIS (2) (pp. 165-170).
- Carreira-Perpinán, M. A. (2015). A review of mean-shift algorithms for clustering. arXiv preprint arXiv:1503.00687.
- Islam, S., & Ahmed, M. (2013). Implementation of image segmentation for natural images using clustering methods. Int J Emerg Technol Adv Eng, 3(3), 175-80.
- Xian, M., Zhang, Y., Cheng, H. D., Xu, F., & Ding, J. (2016). Neutro-connectedness cut. IEEE Transactions on Image Processing, 25(10), 4691-4703.
- Koundal, D., & Sharma, B. (2019). Challenges and future directions in neutrosophic set-based medical image analysis. In Neutrosophic Set in Medical Image Analysis (pp. 313-343). Academic Press.
- J. Shan, H. Cheng, and Y. Wang, ‘A novel segmentation method for breast ultrasound images based on neutrosophic l means clustering’, Med. Phys., vol. 39, no. 9, pp. 5669–5682, 2012.
- Y. Su, Y. Wang, J. Jiao, and Y. Guo, ‘Automatic detection and classification of breast tumors in ultrasonic images using texture and morphological features’, Open Med. Inform. J., vol. 5, no. 1, 2011.
- H. A. Nugroho, M. Rahmawaty, Y. Triyani, and I. Ardiyanto, ‘Neutrosophic and fuzzy C-means clustering for breast ultrasound image segmentation’, presented at the 2017 9th International Conference on Information Technology and Electrical Engineering (ICITEE), 2017, pp. 1–5.
- J. Shan, ‘A fully automatic segmentation method for breast ultrasound images’, 2011, theses and Dissertations. 905
- S. Rajasri and D. R. Gandh, ‘Rule Based Fuzzy Image Segmentation for the Detection of Breast Cancer from Ultrasound Image’, presented at the 2018 International Conference on Intelligent Computing and Communication for Smart World (I2C2SW), 2018, pp. 254–259.
- Y. Feng et al., ‘An adaptive fuzzy C means method utilizing neighboring information for breast tumor segmentation in ultrasound images’, Med. Phys., vol. 44, no. 7, pp. 3752–3760, 2017.
- L. Panigrahi, K. Verma, and B. K. Singh, ‘Hybrid segmentation method based on multi-scale Gaussian kernel fuzzy clustering with spatial bias correction and region-scalable fitting for breast US images’, IET Comput. Vis., vol. 12, no. 8, pp. 1067–1077, 2018.