Semantic-Based K-Means Clustering for IMDB Top 100 Movies
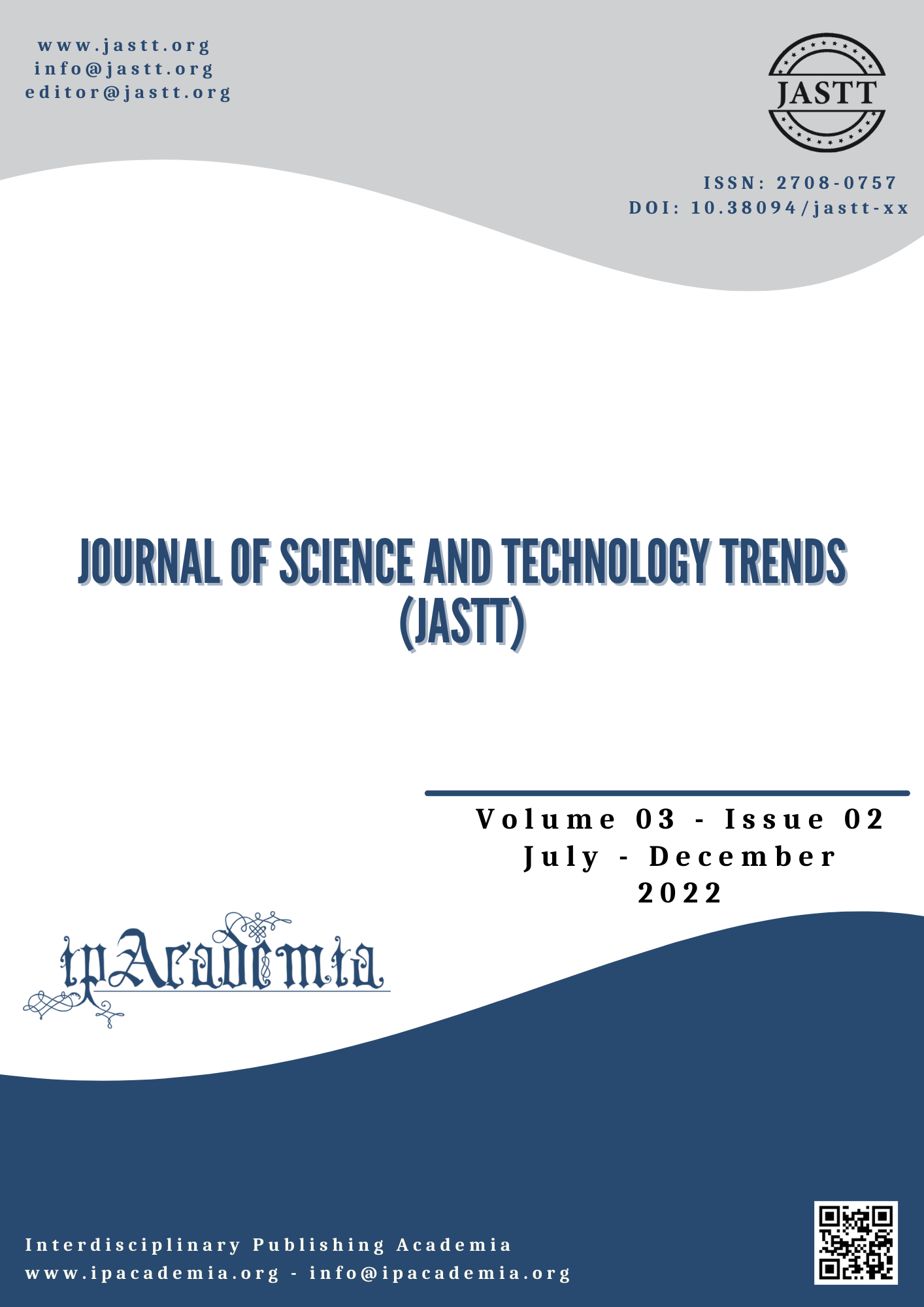
Abstract
Textual documents are growing rapidly through the internet in today’s modern technology era. Electronic structured databases archive offline and online documents, e-mails, webpages, blog and social network posts. Without appropriate ranking and demand clustering when there is classification without any specifics, it is quite difficult to retain and access these documents. K-means is one of the methods that is frequently used for clustering. In terms of determining the proximity of meaning or semantics between data, the distance-based K-means method still has flaws. To get around this issue, semantic similarity can be estimated by measuring the level of similarity between objects in a cluster. This research provides a method for clustering documents based on semantic similarity. The approach is carried out by defining document synopses from the IMDB and Wikipedia databases using the NLTK dictionary, and we provide a semantic-based K-means clustering approach that assesses not only the similarity of the data represented as a vector space model with TFIDF, but also the semantic similarity of the data Precision, recall, and F-measure, we demonstrate how well the semantic-based K-means clustering technique works using experimental findings from the IMDB and Wikipedia top 100 movies datasets.
Keywords
K-means Algorithm, Document Clustering, Semantic Similarity, TF-IDF
Author Biography
Niyaz Salih
References
- S. Mohammed, K. Jacksi, and S. Zeebaree, “A state-of-the-art survey on semantic similarity for document clustering using GloVe and density-based algorithms,” Indonesian Journal of Electrical Engineering and Computer Science, vol. 22, pp. 552–562, Apr. 2021, doi: 10.11591/ijeecs.v22.i1.pp552-562.
- S. AFREEN and D. B. SRINIVASU, “SEMANTIC BASED DOCUMENT CLUSTERING USING LEXICAL CHAINS,” 2017.
- N. M. Salih and K. Jacksi, “State of the art document clustering algorithms based on semantic similarity,” Jurnal Informatika, vol. 14, pp. 58–75, May 2020, doi: 10.26555/jifo.v14i2.a17513.
- R. Ibrahim et al., Clustering Document based on Semantic Similarity Using Graph Base Spectral Algorithm. 2022, p. 259. doi: 10.1109/IICETA54559.2022.9888613.
- I. B. G. Sarasvananda, R. Wardoyo, and A. K. Sari, “The K-Means Clustering Algorithm With Semantic Similarity To Estimate The Cost of Hospitalization,” Indonesian J. Comput. Cybern. Syst., vol. 13, no. 4, Art. no. 4, Oct. 2019, doi: 10.22146/ijccs.45093.
- E. M. B. Nagoudi, J. Ferrero, D. Schwab, and H. Cherroun, “Word Embedding-Based Approaches for Measuring Semantic Similarity of Arabic-English Sentences,” in Arabic Language Processing: From Theory to Practice, vol. 782, Cham: Springer International Publishing, 2018, pp. 19–33. doi: 10.1007/978-3-319-73500-9_2.
- N. M. Salih and K. Jacksi, Semantic Document Clustering using K-means algorithm and Ward’s Method. 2021. doi: 10.1109/ICOASE51841.2020.9436588.
- M. Rafi and M. S. Shaikh, “An improved semantic similarity measure for document clustering based on topic maps.” arXiv, Mar. 17, 2013. doi: 10.48550/arXiv.1303.4087.
- S. Wang and R. Koopman, “Clustering articles based on semantic similarity,” Scientometrics, vol. 111, pp. 1017–1031, 2017, doi: 10.1007/s11192-017-2298-x.
- S. Dhuria, H. Taneja, and K. Taneja, “NLP and ontology based clustering — An integrated approach for optimal information extraction from social web,” in 2016 3rd International Conference on Computing for Sustainable Global Development (INDIACom), Mar. 2016, pp. 1765–1770.
- S. Shehata, “A WordNet-Based Semantic Model for Enhancing Text Clustering,” in 2009 IEEE International Conference on Data Mining Workshops, Dec. 2009, pp. 477–482. doi: 10.1109/ICDMW.2009.86.
- C. Ding, “A Probabilistic Model for Latent Semantic Indexing,” Journal of the American Society for Information Science and Technology, vol. 56, pp. 65–74, Apr. 2005, doi: 10.1002/asi.20148.
- A. Awajan, “Semantic Similarity Based Approach for Reducing Arabic Texts Dimensionality,” International Journal of Speech Technology, Jun. 2015, doi: 10.1007/s10772-015-9284-6.